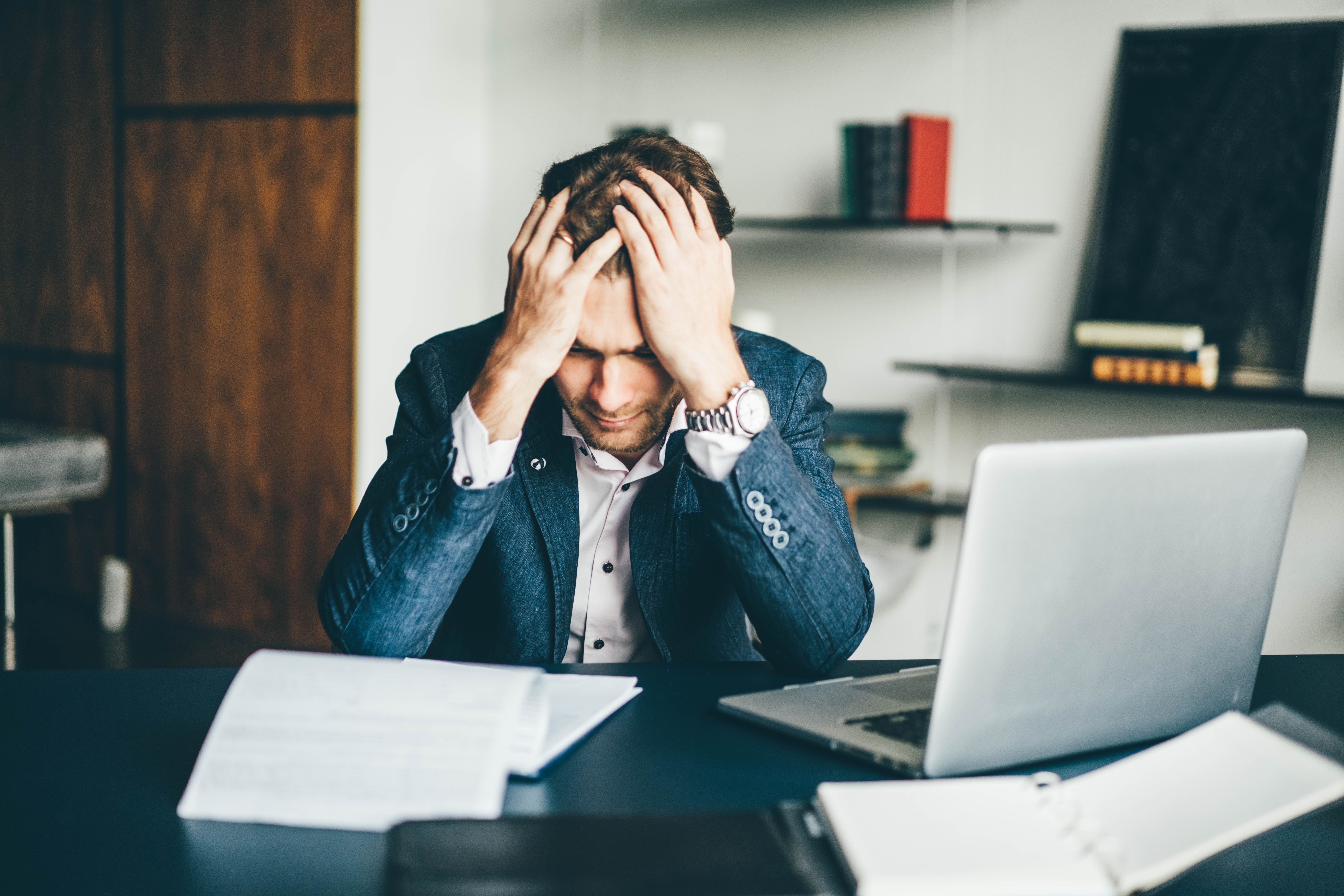
Machine learning model to improve adult ADHD diagnosis
Unmet Need
Attention-deficit hyperactivity disorder (ADHD) is a common neurodevelopmental disorder with a substantial effect on families and society. It is marked by the person’s hyperactivity, inability to keep focus and control impulsive behaviors. Despite the high prevalence of this disorder, standardized and validated approaches for diagnosing ADHD are lacking. Typical adult diagnosis relies on the collection of a large amount of clinical data from multiple sources (patient, observers, family members), along with considerable time on the part of the clinician to integrate and interpret this information. However, in primary care settings, where the large majority of ADHD diagnoses are made, providers do not have the time or resources to collect and analyze this volume of clinical information. This results in an increase of the misdiagnosis probability and subsequent suboptimal treatment for referred patients. Therefore, there is a critical need to help identify which pieces of clinical data contribute most meaningfully to a diagnosis of ADHD and develop methods for efficient diagnosis.
Technology
Duke researchers use machine-learning algorithm to develop predictive models for ADHD diagnosis (and lack thereof) from an existing set of complex clinical data. This algorithm will predict ADHD diagnosis with maximum diagnostic sensitivity, specificity, and predictive power for both positive and negative cases. In addition, it will allow to quantify the importance of various factors to the performance of the model, and to identify data that are essential for ADHD prediction status. This algorithm will therefore guide the efficient use of clinical tools, resulting in increased objectivity and reliability in adult ADHD diagnosis. Furthermore, it will help to decrease the time and resources spent to collect and interpret large amounts of patient data for clinicians to make an accurate diagnosis.
Advantages
- ADHD diagnosis method with great diagnostic sensitivity, specificity, and predictive power.
- Decreases the time and resources spent to collect and interpreted clinical data to make an accurate diagnosis.
- Helps to reduce the number of ADHD misdiagnosis case and resulting inaccurate prescriptions for stimulant medication.